Generative AI to revolutionise the Data-Driven Business
Generative AI will impact across the Transformation life-cycle, and drive innovation in Change Management.
In this latest article on Generative Artificial Intelligence (Generative AI) and its impact on organisations, we explore how Generative AI will shape architecture, programmatic's, service design and strategic delivery, and revolutionise services across business, technical and operational areas. We will explore how it will impact transformation across the life-cycle, and how it could also drive innovation in Change Management.
Revolutionising Complex Business Processes
Generative AI technology has the potential to completely change the way businesses operate, making processes more efficient, accurate and cost-effective.
Generative AI technology is a form of artificial intelligence (AI), which uses natural language processing (NLP) and machine learning (ML) to generate human-like synthetic text or data. Generative AI technology can mimic the way humans write, talk and think, making it an ideal tool for automating complex business processes. Just to give a flavour of the level of automation and capability possible, we have a few examples of where It could be used;-
- Automate tasks such as generating reports, filling out forms and creating emails.
- Automated customer service interaction, to improve quality and experience in supporting customers with their queries and questions.
- Analyse large datasets quickly and accurately, providing valuable insights that can be used by researchers to make informed decisions.
- Automate research tasks such as collecting data from multiple sources and analysing it in order to reach meaningful conclusions.
- Assist researchers identify trends in large datasets that would have been difficult or impossible for human analysts to detect.
Predictive Analytics
In the field of predictive analytics (using data analysis techniques to predict future outcomes or behaviour based on past data), Generative AI can be used to anticipate customer needs before they arise, and make decisions about how to allocate resources, or to respond to changes in demand. For example, Identify changes in consumer demand for products (based on social media, new articles, or related media coverage ) in order to adjust production levels accordingly or develop new products to compensate.
By automating mundane tasks such as data collection or predictive analytics, Generative AI can save both time and resources while providing valuable insights into customers’ needs, as well as being supportive in predicting future trends. As this field continues to evolve, Generative AI will likely become an essential tool for all data or customer focused businesses looking for cost-effective solutions that boost efficiency while maintaining accuracy.
The relationship between Generative AI and BigData
The ability to interpret, evaluate and create new datasets from existing sets, enables solutions utilising predictive analytics, natural language processing (NLP), and image recognition to benefit from further insight, trend and opportunities.
The ability to leverage large volumes of unstructured or semi-structured data for a wide range of applications, coupled with the ability to extract meaningful structured data from unstructured or semi-structured text, can help drive decision support, reporting, analysis, predictions etc . This makes it easier for businesses to gain insights from their data sources, build this insight into their standard processes/operational activities and make better decisions based on the information available.
The ability to “understand” and “infer” enables the integration of disparate data sources into unified sets (for example recognising that Andy Smith, A Smith, and Andrew Smith might all be the same person). By using advanced algorithms, Generative AI can detect patterns in different types of data sources and match them up with corresponding data points in other sources. This enables an integrated view of customers, products, services, process across all areas of business operations, encompassing multiple systems and databases. This can further enable the identification of trends - faster and more accurately than ever before.
Generative AI also has the ability to automate complex tasks like feature engineering (which involves creating features from existing datasets), pattern matching, clustering (grouping similar items together), and anomaly detection (identifying unusual patterns). Generative AI reduces the amount of time needed for (expert) manual processes significantly.
It can also be used to support Data Protection, by applying anonymisation of sensitive information through shielding/masking or transforming. Customer privacy can be maintained while still allowing access to the core information needed for analytics. A secondary benefit being the enhancement of customer trust, and reducing privacy concerns.
Generative AI can provide a key component in big data strategy, but supporting/enabling
- The generation of synthetic datasets,
- Integration of disparate data sources into unified structures,
- Reduction in manual processing time related to big data collection and analysis, and
- Protection of sensitive customer information through automated masking or transformation processes;.
Generative AI as part of Lean Six Sigma
Lean Six Sigma is an integrated process improvement methodology designed to reduce variability and achieve operational excellence. Lean Six Sigma combines the principles of Lean Manufacturing with Six Sigma, which is a set of techniques used to reduce variation and defects in processes. As part of a Lean Six Sigma implementation, organisations must identify process bottlenecks, measure process performance, develop improvement plans, and track progress.
Generative AI technology can be an key enabler in streamlining Lean Six Sigma implementations by providing insights into process variations and identifying potential improvements. For example, Generative AI’s natural language processing (NLP) capabilities can be used to analyse customer feedback data in order to identify pain points within the customer journey. This analysis can then be used to identify areas for improvement and create more customer-centric processes. It can also be used to detect patterns in customer behaviour, in order to identify opportunities for product customisation or personalisation that could drive customer satisfaction. It can help detect chaotic or immature processes, to help identify where problems are occurring (eg How many Sales require an additional interaction with the help desk, How many sales are lost as a result of inaccurate or low-quality information etc).
Generative AI’s machine learning (ML) capabilities applied to production data can support the identification of areas for improvement (such as waste reduction or efficiency optimization). Over time, Generative AI can help organisations to quickly identify opportunities for process improvement (in ‘real time’ ) and develop actionable plans for operational excellence.
And, as part of process improvement, Generative AI can also be used to track progress / maturity of operational excellence, by analysing production data over time and generating insight that highlights process performance trends.
System Development and Application Build
Generative AI can already support system and software build processes, and revolutionise the building and development of new services. It has already demonstrated how it is capable of automatically generating software code, from simple Natural Language. This will reduce the time and cost associated with software development, as well as increase the reliability and accuracy of the code that is generated.
Through the analysis of system requirements, user stories, and other business information, Generative AI can create a code base that is tailored to the needs of the new service. Generative AI will generate data structures, algorithms, and other components that are necessary for the development of the system.
It can also generate code that is optimised for the specific platforms or software environment(s), making the development process more efficient and cost effective. With the code base created, Generative AI then is able to use its machine learning algorithm to generate the optimised software, specific to the needs and environment.
With this understanding of what the goal/project is about, Generative AI can also then generate the unit tests for each component to ensure that the code is reliable and meets the needs.
With the generated system, it is then able to run simulations against the solution to the logic and ensure the code meets the requirements (emulating how the system will work in a real-world scenario). A by-product is that the developer is able to quickly see how the application will work in real scenarios, and that it operates as the user is likely to expect. In the event that the code fails the simulation, Generate AI can then suggest(make) the changes to the code either resolve or make the implementation more effective.
Finally, the necessary tasks of reporting, commenting, auditing as well as the changes that were made to the code, can all be created automatically, providing confidence to third parties of the robustness and quality of the solution.
Generate AI is a powerful tool that has the potential to revolutionise the process of software development. By automating code generation, Generative AI can reduce the time and cost associated with software development, ensuring that the development is tailored to the (target) environment. Ultimately this will make the whole engineering development cycle more efficient and effective, ensuring that the solution is more reliable and accurate.
Improving quality and performance of complex Knowledge systems and Processes
Generative AI technology can be used to help simplify Knowledge based solutions, by being able to interpret the information and generate automated insights. By leveraging natural language processing (NLP) and machine learning (ML), it can identify patterns in data that would otherwise be difficult to detect. This will subsequently support the automation of the decision-making process(es) ( for example recommending products or services based on customer preferences). While increasing accuracy, improving response and quality of insight, it will also have the effect of dramatically improving customer experience.
Processes like report generation, problem solving, decision making, expert system design etc can all be improved both in terms of accuracy, quality and speed through applying Generative AI capabilities across data sets. For example, AI system can interpret financial reports, emails and data, combining and analysing the data to identify patterns that indicate fraudulent activity, or to recommend strategies for reducing costs based on past trends.
By leveraging machine learning algorithms such as deep learning networks or reinforcement learning models, Generative AI systems can support the detection of discrepancies in data sets that would otherwise go unnoticed. This helps organisations quickly identify potential issues before they become costly mistakes.
Conclusion
Generative AI technology will have a significant impact on the profitability of successful data-driven businesses. Businesses that rely heavily on information processing are likely to benefit the most from using this technology in order to improve not any the capabilities, reduce their costs, improve their quality of service, but be able to take advantage of new service and products that are tailored to the needs of their users.
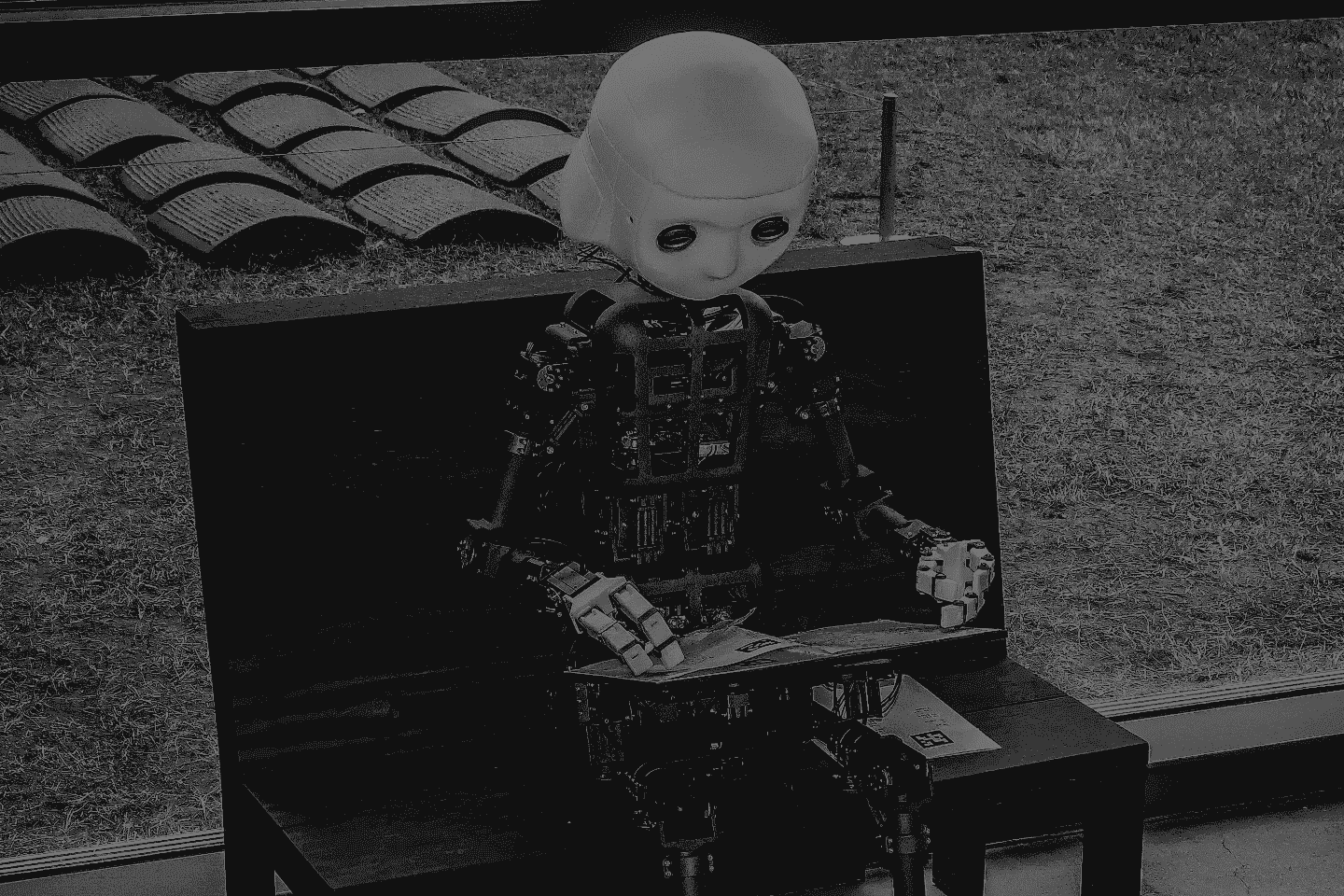